Annotation Tools for Machine Learning: Empowering Your Data Journey
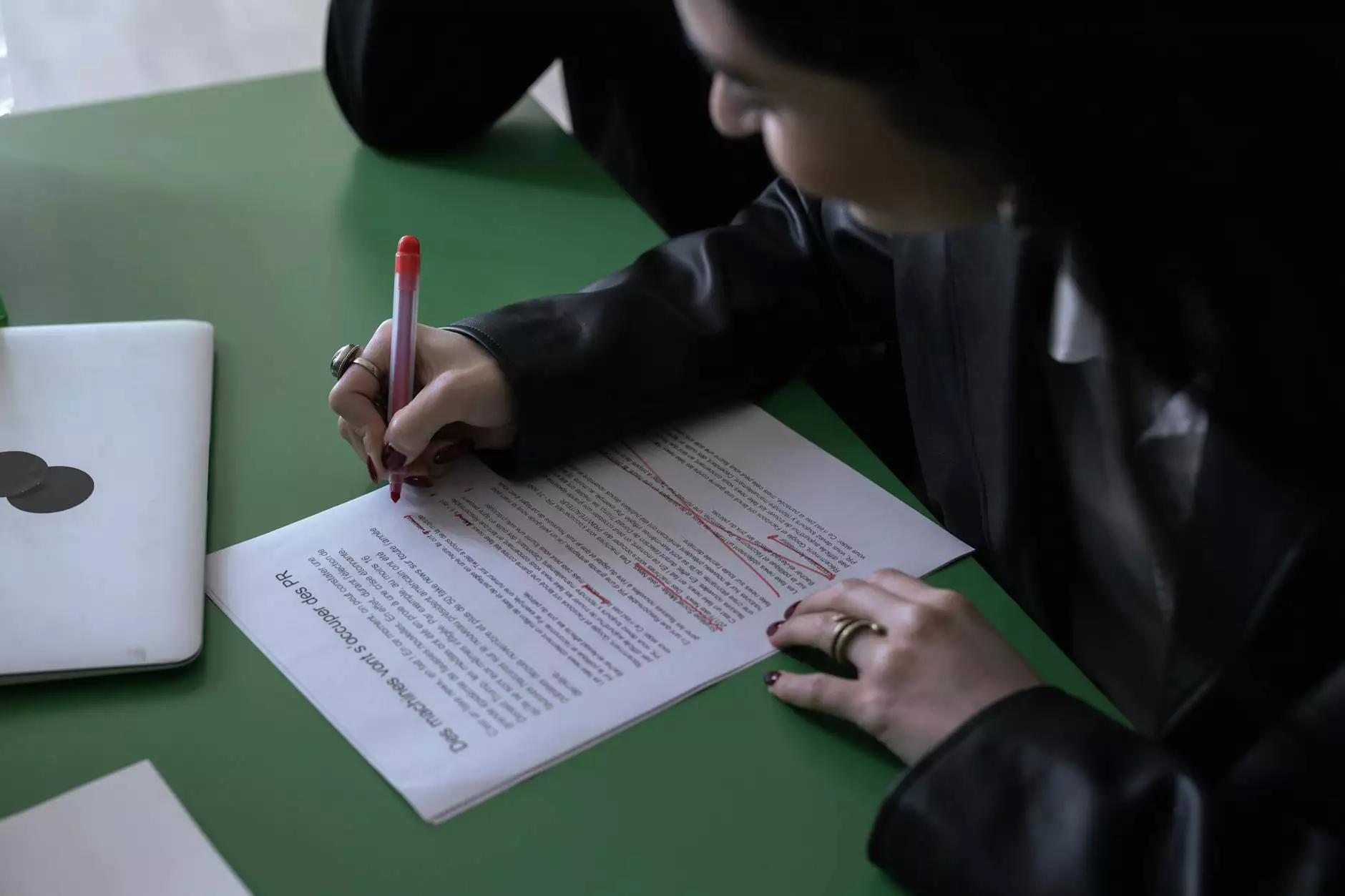
The rapid evolution of machine learning has transformed industries, driving innovation and creating new opportunities. One of the foundational elements of successful machine learning projects is data annotation. This is where annotation tools for machine learning come into play. In this comprehensive guide, we will explore the significance of these tools, their features, and how they can streamline your workflow, enhance model accuracy, and ultimately boost your business performance.
The Importance of Data Annotation in Machine Learning
Data annotation involves label identification, categorization, and contextualizing raw data. It is essential for training machine learning models, as the quality and quantity of annotated data directly influence model performance. Here are several reasons why data annotation is significant:
- Model Accuracy: High-quality annotated data enhances the precision of machine learning models, allowing them to make better predictions.
- Understanding Context: Proper annotation helps algorithms understand the nuances of data, such as distinguishing between different categories or identifying sentiment.
- Scalability: As businesses grow, the volume of data increases. Efficient annotation tools allow for scalable data management and processing.
- Time Efficiency: Automated annotation tools can drastically reduce the time required to label data, speeding up project timelines.
Key Features of Annotation Tools for Machine Learning
Investing in the right annotation tools for machine learning can greatly enhance your data processing efforts. Below are critical features to consider:
User-Friendly Interface
A user-friendly interface can significantly reduce the learning curve for your team. Tools with intuitive dashboards allow for easy navigation and interaction with data.
Support for Multiple Data Types
Different machine learning projects require various types of data (text, images, audio, video). A robust annotation tool should support multiple formats, making it versatile for different applications.
Collaboration Features
Collaboration tools enable teams to work together efficiently. Look for features that support commenting, task assignments, and progress tracking, ensuring that everyone stays on the same page.
Automation Capabilities
With advancements in artificial intelligence, some annotation tools now offer automation features. These capabilities can expedite the labeling process and reduce the manual workload, allowing data scientists to focus on model development.
Quality Control Mechanisms
Ensuring data quality is paramount. Look for tools that include quality control features such as inter-annotator agreement metrics and validation checks to maintain high data standards.
Top Annotation Tools for Machine Learning in 2023
Numerous annotation tools are available in the market. Here’s a list of some of the most reliable and powerful options for machine learning practitioners:
1. Labelbox
Labelbox offers a comprehensive platform for data labeling suitable for images, videos, and text. Its intuitive interface and collaboration capabilities make it a popular choice for enterprises looking to streamline their ML pipelines.
2. Supervisely
Designed mainly for computer vision applications, Supervisely provides advanced tools for image and video annotation. Its powerful automation features help in speeding up the annotation process significantly.
3. Prodigy
Prodigy is a scriptable annotation tool that enhances the manual annotation process by focusing on active learning. Its flexibility allows teams to create customized labeling workflows that suit their project's unique needs.
4. VGG Image Annotator (VIA)
This open-source tool is excellent for image annotation and offers features like regional dragging and polygon annotations. It’s particularly favored for research and smaller-scale projects.
5. Amazon SageMaker Ground Truth
A versatile tool from Amazon, SageMaker Ground Truth enables users to build highly accurate training datasets quickly. Its integration with other AWS services is beneficial for businesses already within the Amazon ecosystem.
How to Choose the Right Annotation Tool for Your Needs
Selecting the best annotation tools for machine learning can be a daunting task given the vast number of solutions available. Here are several considerations to guide your choice:
- Project Requirements: Understand the specific needs of your ML project. Different projects have different data types and complexities.
- Budget: Consider your budget constraints. Some tools offer free versions, while others may require expensive subscriptions.
- Integration Capabilities: Ensure that the tool you select can seamlessly integrate with your existing tech stack and workflow.
- Scalability: Choose a solution that can grow with your business and adapt to increasing data volumes and complexities.
- User Reviews and Support: Research user experiences and customer support options. Quality support can save you time and frustrations down the line.
Best Practices for Data Annotation
Once you have selected your annotation tool, follow these best practices to maximize its effectiveness:
1. Define Clear Guidelines
Provide detailed guidelines to annotators to ensure consistency and accuracy in the annotations. This includes defining categories, quality standards, and specific instructions for ambiguous cases.
2. Utilize Active Learning
Integrate active learning into your workflow to prioritize difficult-to-label examples. This approach can enhance model performance by focusing on areas where the model struggles.
3. Iterate and Improve
Regularly review and update your annotation processes and guidelines. Collect feedback from your annotators and data scientists to continually enhance your workflows.
4. Maintain a Balanced Dataset
Ensure that your annotated data reflects a balanced representation of all categories. This prevents biases in your machine learning model and leads to more reliable predictions.
5. Invest in Quality Control
Implement quality control measures to validate annotations. Regular audits and feedback loops can help maintain the integrity of your datasets.
Conclusion: The Future of Machine Learning Annotation
As machine learning technology continues to evolve, the importance of high-quality data annotation will only grow. Annotation tools for machine learning are pivotal in this landscape, enabling businesses to harness their data effectively and drive innovation. From enhancing model performance to improving operational efficiencies, these tools are indispensable assets for any organization looking to thrive in the age of AI.
In selecting the right tools for your projects, consider the specific features, usability, and scalability that align with your goals. By implementing best practices, you can not only improve your current workflows but also set your organization up for future success in a competitive marketplace. Embrace the power of data annotation as the catalyst for your machine learning initiatives, ensuring your models are equipped with the best training data available.