Unlocking Business Potential with Data Labeling
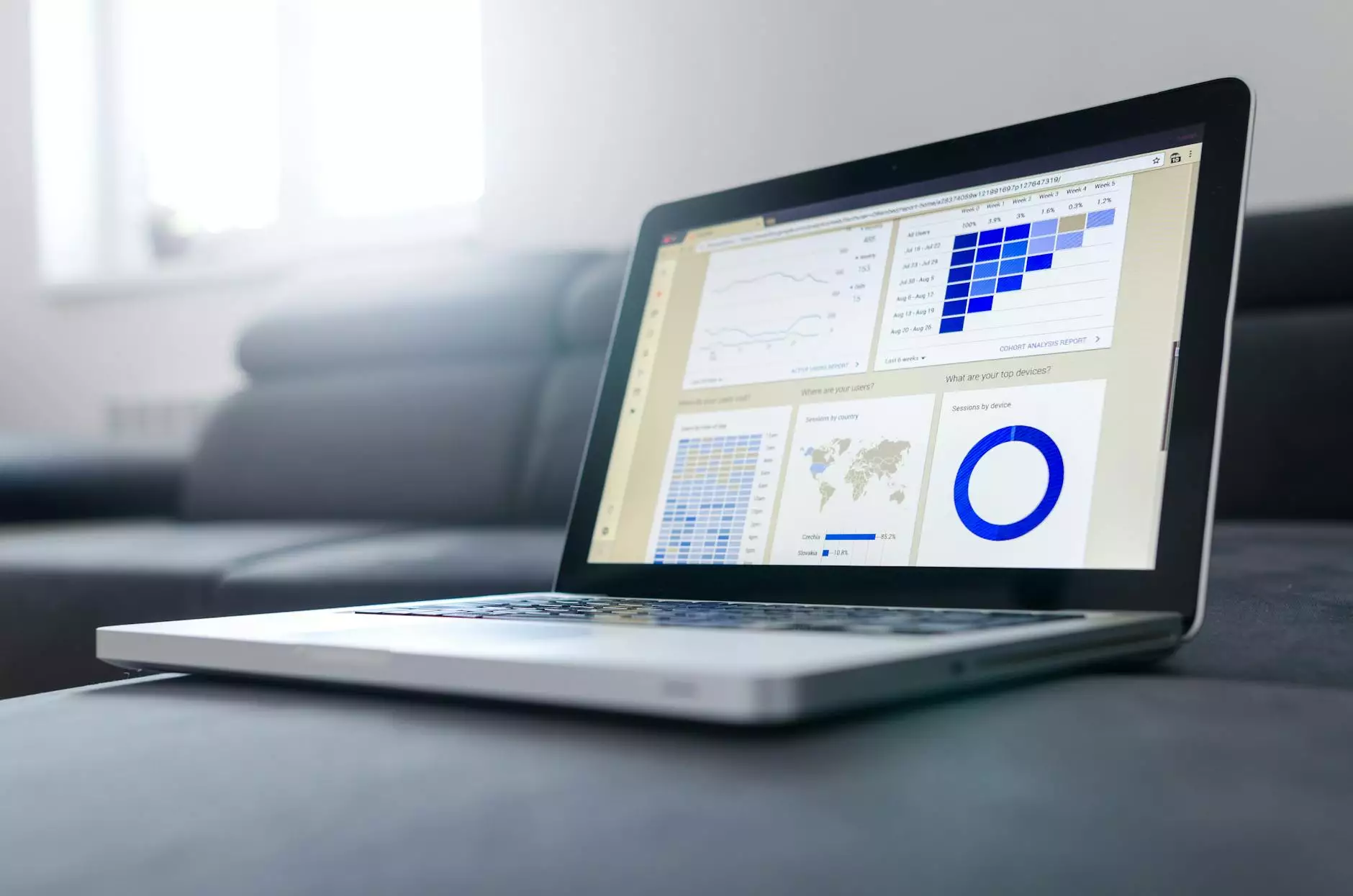
Data labeling has become a critical component of the business landscape, particularly as organizations increasingly rely on data-driven decision-making. In a world where information is power, understanding how to effectively label and categorize data can elevate business strategies, improve efficiencies, and ensure compliance with regulatory standards. This article will delve into the intricacies of data labeling, its significance, and how leveraging it through tools and platforms can lead to substantial business benefits.
The Importance of Data Labeling in Today's Business Environment
As businesses drown in vast quantities of data, the need for accurate and efficient data annotation has never been more crucial. Without well-labeled data, machine learning models can fail to recognize patterns, leading to poor decision-making. A well-executed data labeling strategy can transform raw data into organized, useful insights.
- Enhancing Machine Learning Accuracy: Properly labeled data is essential for training machine learning models. The more accurate and comprehensive the labels, the better the model's predictive capabilities.
- Improving Decision-Making: Business decisions grounded in well-analyzed data lead to better outcomes. Data labeling ensures that analysts can extract meaningful insights from complex datasets.
- Facilitating Compliance: Many industries are subject to stringent regulations regarding data handling. Accurate labeling helps maintain compliance with legal obligations.
Key Components of an Effective Data Labeling Strategy
Implementing an effective data labeling strategy requires careful consideration of several key components:
1. Choose the Right Data Annotation Tool
The selection of a suitable data annotation tool is critical for the success of your labeling efforts. A good tool should be user-friendly, scalable, and capable of handling various data types including text, images, and videos. For example, KeyLabs.ai offers a versatile platform that provides businesses with innovative solutions for data labeling.
2. Train a Skilled Workforce
No matter how sophisticated the tools are, human oversight is necessary for achieving high-quality results. Providing adequate training for the workforce involved in data labeling can greatly enhance the accuracy of the annotated data. Key training areas include:
- Understanding the specific labeling requirements
- Interpretation of data context
- Data privacy and compliance training
3. Implement Quality Control Mechanisms
Quality assurance in data labeling cannot be overlooked. Setting up a multi-stage review process can help identify errors and inconsistencies before the data is used for model training. Regular audits and feedback loops can also maintain standards.
Data Labeling Methods: Finding the Best Fit for Your Business
There are various data labeling methods, and selecting the most appropriate one depends on your business needs:
- Manual Labeling: This method involves human annotators reviewing and labeling data. It is time-consuming but produces high-quality results.
- Automated Labeling: Automated techniques use algorithms to label data quickly. However, the accuracy may vary based on the sophistication of the algorithms.
- Semi-Automated Labeling: This is a hybrid approach that combines both manual and automated methods, often striking a balance between speed and accuracy.
The Role of Data Annotation Platforms in Business
Data annotation platforms simplify the complexities associated with data labeling. They provide businesses access to tools that automate part of the labeling process and facilitate collaborative efforts across teams. Some functionalities to look for in a robust data annotation platform include:
Collaboration Features
Data labeling often requires teamwork from various departments. A good platform enables real-time collaboration, allowing multiple users to work on the same dataset simultaneously, thus speeding up the process.
Flexibility and Scalability
As businesses grow, so does their data. An ideal data annotation platform should accommodate increasing data volumes and types, without compromising quality.
Integration Capabilities
Integration with existing data management systems is essential to streamline workflows and ensure that labeled data can be easily accessed and utilized. This aids in maintaining continuity in data operations.
Business Use Cases for Data Labeling
Data labeling can be applied across various sectors, each harnessing its potential uniquely:
1. Retail and E-commerce
Retailers use data labeling for product classification, sentiment analysis, and customer behavior prediction. By understanding their customer base better, they can personalize marketing campaigns and optimize inventory management.
2. Healthcare
Healthcare organizations leverage data labeling for medical image analysis, electronic health records, and clinical trial data. Properly labeled data enables precise diagnostics and patient care strategies.
3. Autonomous Vehicles
Data labeling plays a vital role in the development of self-driving cars. Annotated images from the car's environment help in training models to recognize and react to various traffic conditions and obstacles.
Measuring the Impact of Data Labeling on Business Outcomes
Understanding the return on investment from data labeling efforts is essential. Businesses can measure impact by:
- Tracking Model Accuracy: Analyze how improvements in data labeling correlate with the accuracy of machine learning models.
- Assessing Operational Efficiency: Monitor time savings and resource allocation efficiency due to effective data management practices.
- Evaluating Decision-Making Quality: Track business outcomes following enhanced data-driven strategies.
Conclusion: The Future of Data Labeling in Business
As we move towards an increasingly data-centric future, the data labeling landscape will continue to evolve. With advancements in artificial intelligence and machine learning, the methodologies for data annotation will become even more sophisticated, making it essential for businesses to stay ahead of the curve.
By investing in comprehensive data labeling strategies, organizations can not only improve their operational efficiencies but also gain a competitive edge in the market. The tools and platforms available today, such as those offered by KeyLabs.ai, empower businesses to harness the full potential of their data and drive innovation.
In conclusion, prioritizing data labeling is not just about improving machine learning outcomes; it's about transforming business operations and ensuring sustainable growth in the digital age.